Abstract
Classical stochastic model predictive control (SMPC) methods assume that the true probability distribution of uncertainties in controlled systems is provided in advance. However, in real-world systems, only partial distribution information can be acquired for SMPC. The discrepancy between the true distribution and the distribution assumed can result in sub-optimality or even infeasibility of the system. To address this, we present a novel distributionally robust data-driven MPC scheme to control stochastic nonlinear systems. We use distributionally robust constraints to bound the violation of the expected state-constraints under process disturbance. Sequential linearization is performed at each sampling time to guarantee that the system’s states comply with constraints with respect to the worst-case distribution within the Wasserstein ball centered at the discrete empirical probability distribution. Under this distributionally robust MPC scheme, control laws can be efficiently derived by solving a conic program. The competence of this scheme for disturbed nonlinear systems is demonstrated through two case studies.
Publication
IFAC-PapersOnLine
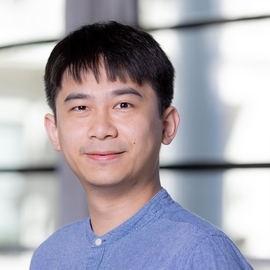
Data-Driven Distributionally Robust Control
Zhengang is a PhD candidate at Imperial College London. His PhD research is about data-driven distributionally robust model predictive control.Prior to his PhD research, he holds a Diplom-Ingenieur in Mechatronics from Technische Universität Dresden. His research interests include data-driven optimal control, scientific machine learning, and distributionally robust optimisation.
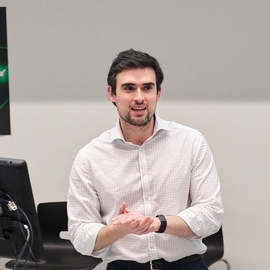
Principal Investigator of OptiML
Antonio del Rio Chanona is the head of the Optimisation and Machine Learning for Process Systems Engineering group based in thee Department of Chemical Engineering, as well as the Centre for Process Systems Engineering at Imperial College London. His work is at the forefront of integrating advanced computer algorithms from optimization, machine learning, and reinforcement learning into engineering systems, with a particular focus on bioprocess control, optimization, and scale-up. Dr. del Rio Chanona earned his PhD from the Department of Chemical Engineering and Biotechnology at the University of Cambridge, where his outstanding research earned him the prestigious Danckwerts-Pergamon award for the best PhD dissertation of 2017. He completed his undergraduate studies at the National Autonomous University of Mexico (UNAM), which laid the foundation for his expertise in engineering.