Abstract
Online Feedback Optimization is a method used to steer the operation of a process plant to its optimal operating point without explicitly solving a nonlinear constrained optimization problem. This is achieved by leveraging a linear plant model and feedback from measurements. However the presence of plant-model mismatch leads to suboptimal results when using this approach. Learning the plant-model mismatch enables Online Feedback Optimization to overcome this shortcoming. In this work we present a novel application of Online Feedback Optimization with online model adaptation using Gaussian process regression. We demonstrate our approach with a realistic load sharing problem in a compressor station with parametric and structural plant-model mismatch. We assume imperfect knowledge of the compressor maps and design an Online Feedback Optimization controller that minimizes the compressor station power consumption. In the evaluated scenario, imperfect knowledge of the plant leads to a 5% increase in power consumption compared to the case with perfect knowledge. We demonstrate that Online Feedback Optimization with model adaptation reduces this increase to only 0.8%, closely approximating the case of perfect knowledge of the plant, regardless of the type of mismatch.
Publication
Journal of Process Control
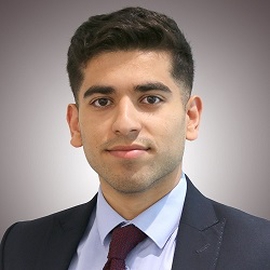
Head of Innovation at PE Limited, former PhD student at OptiML (2021-2025)
Akhil is a PhD candidate working at the intersection of machine learning, optimization and control. He has a proven track record in practical problem solving across diverse industries and domains with research contributions published in various conferences and journals. He graduated with a first class/distinction from the University of Strathclyde in Chemical and Process Engineering and worked as a scientific software developer and engineering consultant before commencing his doctoral studies. Akhil is a member of the Autonomous Industrial Systems Lab supervised by Mehmet Mercangoz and he is co-supervised by Antonio del Rio Chanona.
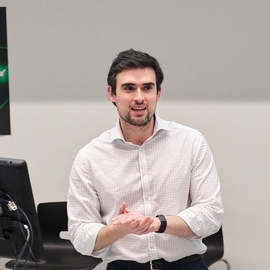
Principal Investigator of OptiML
Antonio del Rio Chanona is the head of the Optimisation and Machine Learning for Process Systems Engineering group based in thee Department of Chemical Engineering, as well as the Centre for Process Systems Engineering at Imperial College London. His work is at the forefront of integrating advanced computer algorithms from optimization, machine learning, and reinforcement learning into engineering systems, with a particular focus on bioprocess control, optimization, and scale-up. Dr. del Rio Chanona earned his PhD from the Department of Chemical Engineering and Biotechnology at the University of Cambridge, where his outstanding research earned him the prestigious Danckwerts-Pergamon award for the best PhD dissertation of 2017. He completed his undergraduate studies at the National Autonomous University of Mexico (UNAM), which laid the foundation for his expertise in engineering.