Abstract
One of the main hurdles in the computer-aided design and optimization of industrial bioprocesses is the limited capability of models to accurately represent biosystems. On one hand, mechanistic models have drawbacks in terms of expressing all potential biomechanisms. On the other hand, data-driven models have limited extrapolation ability. Thus, hybrid models may represent the best of both worlds. However, it is challenging to build a hybrid model that can accurately balance its data-driven and first principles components, hence allowing for extrapolation ability and accuracy. In this work, we propose a framework for the development of hybrid models that includes a mechanistic backbone for its construction and judiciously adds the data-driven components. Statistical methods e.g., Bayesian Information Criterion (BIC), Akaike Information Criterion (AIC) and Hannan Quinn Criterion (HQC), are employed to choose the statistically best hybrid model structure. The proposed framework is tested on a simplified microalgae cultivation case study and shows good prediction capabilities under different noise levels, especially by applying BIC for hybrid model selection.
Publication
Computer Aided Chemical Engineering
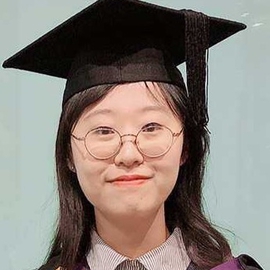
Hybrid modeling for bioprocesses - merging first principles and machine learning
Haiting is a PhD candidate specializing in Digital Twinning for bioprocesses, incorporating hybrid and data-driven models. Her research emphasizes machine learning applications in surrogate modelling, time series prediction, and reinforcement learning for process modelling, scheduling, and optimization in bioengineering. This work has led to a novel framework that merges kinetic modelling with machine learning to optimize dynamic systems. Before her PhD, she was an undergraduate student at Dalian University of Technology and University of Manchester in Chemical Engineering.
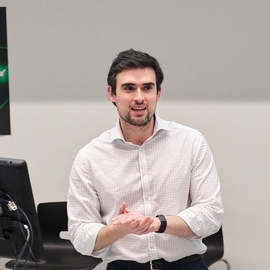
Principal Investigator of OptiML
Antonio del Rio Chanona is the head of the Optimisation and Machine Learning for Process Systems Engineering group based in thee Department of Chemical Engineering, as well as the Centre for Process Systems Engineering at Imperial College London. His work is at the forefront of integrating advanced computer algorithms from optimization, machine learning, and reinforcement learning into engineering systems, with a particular focus on bioprocess control, optimization, and scale-up. Dr. del Rio Chanona earned his PhD from the Department of Chemical Engineering and Biotechnology at the University of Cambridge, where his outstanding research earned him the prestigious Danckwerts-Pergamon award for the best PhD dissertation of 2017. He completed his undergraduate studies at the National Autonomous University of Mexico (UNAM), which laid the foundation for his expertise in engineering.