Abstract
Most optimization problems in engineering can be formulated as ‘expensive’ black box problems whose solutions are limited by the number of function evaluations. Frequently, engineers develop accurate models of physical systems that are differentiable and/or cheap to evaluate. These models can be solved efficiently, and the solution transferred to the real system. In the absence of gradient information or cheap-to-evaluate models, one must resort to efficient optimization routines that rely only on function evaluations. Creating a model can itself be considered part of the expensive black box optimization process. In this work, we investigate how perceived state-of-the-art derivative-free optimization (DFO) algorithms address different instances of these problems in process engineering. On the algorithms side, we benchmark both model-based and direct-search DFO algorithms. On the problems side, the comparisons are made on one mathematical optimization problem and five chemical engineering applications: model-based design of experiments, flowsheet optimization, real-time optimization, self-optimizing reactions, and controller tuning. Various challenges are considered such as constraint satisfaction, uncertainty, problem dimension and evaluation cost. This work bridges the gap between the derivative-free optimization and process systems literature by providing insight into the efficiency of data-driven optimization algorithms in the process systems domain to advance the digitalization of the chemical and process industries.
Publication
Chemical Engineering Science
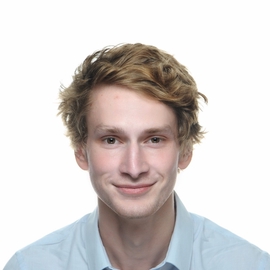
Data-Driven Optimization for the Integration of Interconnected Process Systems
Final-year PhD candidate working at the intersection of optimization and Machine Learning. I investigate how data-driven techniques can aid the optimization of integrated manufacturing and supply chain systems, with a focus on black-box optimization, optimization with embedded neural networks, and Reinforcement Learning for combinatorial optimization. By collaborating with industry, I ensure my case studies are industrially relevant and that my algorithms respect the organizational and business considerations of the process industries.
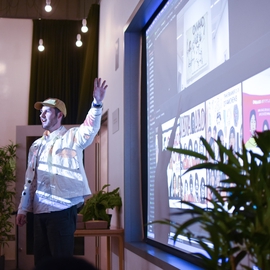
Applied Data-Driven Optimisation
I am a PhD student at Imperial College London & 2023 Enrichment student at the Alan Turing Institute. I have a background in Chemical Engineering and still enjoy teaching labs at Imperial College. Alongside my work in process systems engineering, I am affiliated with Winchester School of Art producing installations with the Tate on the intersection between AI and art. My interests include Bayesian optimisation, human-in-the-loop machine learning, cricket 🏏, and darts 🎯.
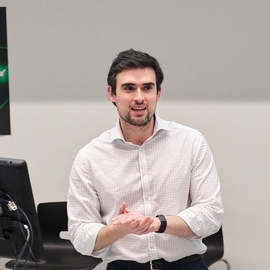
Principal Investigator of OptiML
Antonio del Rio Chanona is the head of the Optimisation and Machine Learning for Process Systems Engineering group based in thee Department of Chemical Engineering, as well as the Centre for Process Systems Engineering at Imperial College London. His work is at the forefront of integrating advanced computer algorithms from optimization, machine learning, and reinforcement learning into engineering systems, with a particular focus on bioprocess control, optimization, and scale-up. Dr. del Rio Chanona earned his PhD from the Department of Chemical Engineering and Biotechnology at the University of Cambridge, where his outstanding research earned him the prestigious Danckwerts-Pergamon award for the best PhD dissertation of 2017. He completed his undergraduate studies at the National Autonomous University of Mexico (UNAM), which laid the foundation for his expertise in engineering.