Abstract
Planning, scheduling, and control typically constitute separate decision-making units within chemical companies. Traditionally, their integration is modelled sequentially, but recent efforts prioritize lower-level feasibility and optimality, leading to large-scale, potentially multi-level, hierarchical formulations. Data-driven techniques, like optimality surrogates or derivative-free optimization, become essential in addressing ensuing tractability challenges. We demonstrate a step-by-step workflow to find a tractable solution to a tri-level formulation of a multi-site, multi-product planning-scheduling-control case study. We discuss solution tractability-accuracy trade-offs and scaling properties for both methods. Despite individual improvements over conventional heuristics, both approaches present drawbacks. Consequently, we synthesize our findings into a methodology combining their strengths. Our approach remains agnostic to the level-specific formulations when the linking variables are identified and retains the heuristic sequential solution as fallback option. We advance the field by leveraging parallelization, hyperparameter tuning, and a combination of off- and on-line computation, to find tractable solutions to more accurate multi-level formulations.
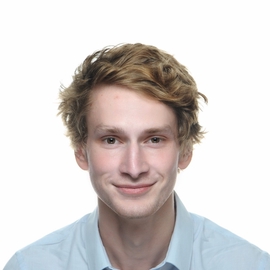
Data-Driven Optimization for the Integration of Interconnected Process Systems
Final-year PhD candidate working at the intersection of optimization and Machine Learning. I investigate how data-driven techniques can aid the optimization of integrated manufacturing and supply chain systems, with a focus on black-box optimization, optimization with embedded neural networks, and Reinforcement Learning for combinatorial optimization. By collaborating with industry, I ensure my case studies are industrially relevant and that my algorithms respect the organizational and business considerations of the process industries.
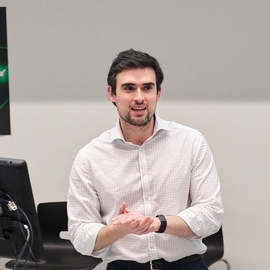
Principal Investigator of OptiML
Antonio del Rio Chanona is the head of the Optimisation and Machine Learning for Process Systems Engineering group based in thee Department of Chemical Engineering, as well as the Centre for Process Systems Engineering at Imperial College London. His work is at the forefront of integrating advanced computer algorithms from optimization, machine learning, and reinforcement learning into engineering systems, with a particular focus on bioprocess control, optimization, and scale-up. Dr. del Rio Chanona earned his PhD from the Department of Chemical Engineering and Biotechnology at the University of Cambridge, where his outstanding research earned him the prestigious Danckwerts-Pergamon award for the best PhD dissertation of 2017. He completed his undergraduate studies at the National Autonomous University of Mexico (UNAM), which laid the foundation for his expertise in engineering.