Abstract
Optimizing complex reactor geometries is vital to promote enhanced efficiency. We present a framework to solve this nonlinear, computationally expensive, and derivative-free problem. Gaussian processes are used to learn a multi-fidelity model of reactor simulations correlating multiple continuous mesh fidelities. The search space of reactor geometries is explored through lower fidelity simulations, evaluated based on a weighted acquisition function, trading off information gain with cost. Within our framework, DARTS, we derive a novel criteria for dictating optimization termination, ensuring a high fidelity solution is returned before budget is exhausted. We investigate the design of helical-tube reactors under pulsed-flow conditions, which have demonstrated outstanding mixing characteristics. To validate our results, we 3D print and experimentally validate the optimal reactor geometry, confirming mixing performance. Our approach is applicable to a broad variety of expensive simulation-based optimization problems, enabling the design of novel parameterized chemical reactors.
Publication
Computers & Chemical Engineering
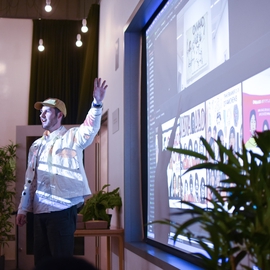
Applied Data-Driven Optimisation
I am a PhD student at Imperial College London & 2023 Enrichment student at the Alan Turing Institute. I have a background in Chemical Engineering and still enjoy teaching labs at Imperial College. Alongside my work in process systems engineering, I am affiliated with Winchester School of Art producing installations with the Tate on the intersection between AI and art. My interests include Bayesian optimisation, human-in-the-loop machine learning, cricket 🏏, and darts 🎯.
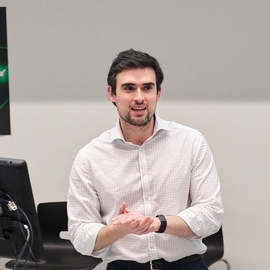
Principal Investigator of OptiML
Antonio del Rio Chanona is the head of the Optimisation and Machine Learning for Process Systems Engineering group based in thee Department of Chemical Engineering, as well as the Centre for Process Systems Engineering at Imperial College London. His work is at the forefront of integrating advanced computer algorithms from optimization, machine learning, and reinforcement learning into engineering systems, with a particular focus on bioprocess control, optimization, and scale-up. Dr. del Rio Chanona earned his PhD from the Department of Chemical Engineering and Biotechnology at the University of Cambridge, where his outstanding research earned him the prestigious Danckwerts-Pergamon award for the best PhD dissertation of 2017. He completed his undergraduate studies at the National Autonomous University of Mexico (UNAM), which laid the foundation for his expertise in engineering.