Abstract
Model-free reinforcement learning has been recently investigated for use in chemical process control. Through the iterative creation of an approximate process model, control actions are able to be explored and optimal policies generated. Typically, this approximate process model has taken the form of a neural network that is continuously updated. However when small quantities of historical data are available, for example in novel processes, neural networks tend to over-fit to data providing poor performance. In this paper Gaussian processes are used as a method of function approximation to describe the action-value function of a non-isothermal semi-batch reactor. Through the use of analytical uncertainty obtained from Gaussian process predictions, trade off between exploration and exploitation is enabled, allowing for efficient generation of effective policies. Importantly Gaussian processes also enable probabilistic constraint violation to be modelled, ensuring safe constraint satisfaction throughout the learning procedure. On application to the in-silico case study, a safe, effective policy was generated utilising only 100 evaluations of process trajectory with no prior knowledge of the process dynamics. A result that would require significantly more trajectory evaluations when compared to a neural network based approach.
Publication
IFAC-PapersOnLine
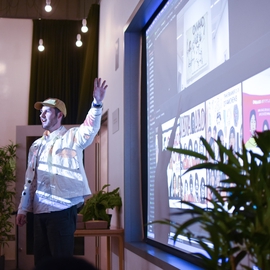
Applied Data-Driven Optimisation
I am a PhD student at Imperial College London & 2023 Enrichment student at the Alan Turing Institute. I have a background in Chemical Engineering and still enjoy teaching labs at Imperial College. Alongside my work in process systems engineering, I am affiliated with Winchester School of Art producing installations with the Tate on the intersection between AI and art. My interests include Bayesian optimisation, human-in-the-loop machine learning, cricket 🏏, and darts 🎯.
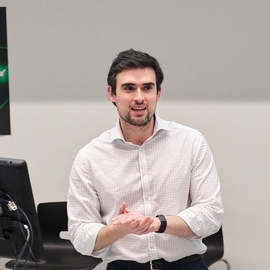
Principal Investigator of OptiML
Antonio del Rio Chanona is the head of the Optimisation and Machine Learning for Process Systems Engineering group based in thee Department of Chemical Engineering, as well as the Centre for Process Systems Engineering at Imperial College London. His work is at the forefront of integrating advanced computer algorithms from optimization, machine learning, and reinforcement learning into engineering systems, with a particular focus on bioprocess control, optimization, and scale-up. Dr. del Rio Chanona earned his PhD from the Department of Chemical Engineering and Biotechnology at the University of Cambridge, where his outstanding research earned him the prestigious Danckwerts-Pergamon award for the best PhD dissertation of 2017. He completed his undergraduate studies at the National Autonomous University of Mexico (UNAM), which laid the foundation for his expertise in engineering.