Abstract
Light attenuation is a primary challenge limiting the upscaling of photobioreactors for sustainable bio-production. One key to this challenge, is to model and optimise the light/dark cycles so that cells within the dark region can be frequently transferred to the light region for photosynthesis. Therefore, this study proposes the first mechanistic model to integrate the light/dark cycle effects into biomass growth kinetics. This model was initially constructed through theoretical derivation based on the intracellular reaction kinetics, and was subsequently modified by embedding a new parameter, effective light coefficient, to account for the effects of culture mixing. To generate in silico process data, a new multiscale reactive transport modelling strategy was developed to couple fluid dynamics with biomass growth kinetics and light transmission. By comparing against previous experimental and computational studies, the multiscale model shows to be of high accuracy. Based on its simulation result, an original correlation was proposed to link effective light coefficient with photobioreactor gas inflow rate; this has not been done before. The impact of this study is that by using the proposed mechanistic model and correlation, we can easily control and optimise photobioreactor gas inflow rates to alleviate light attenuation and maintain a high biomass growth rate.
Publication
Biotechnology and Bioengineering
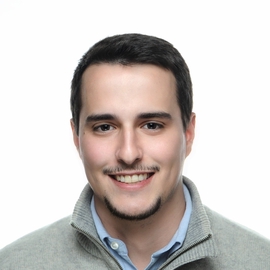
Automated Knowledge Discovery Methods in Reaction Engineering
Miguel Carvalho is a chemical engineering PhD candidate at Imperial College London, affiliated with the EPSRC CDT in Next Generation Synthesis and Reaction Technology. He earned an MEng in chemical engineering from The University of Manchester (2021) and an MRes in advanced molecular synthesis from Imperial College London (2022). His research intersects data science, chemical engineering, and chemistry, concentrating on algorithmic solutions for automated knowledge discovery in reaction engineering and catalysis.
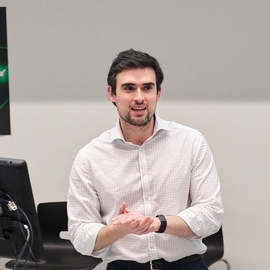
Principal Investigator of OptiML
Antonio del Rio Chanona is the head of the Optimisation and Machine Learning for Process Systems Engineering group based in thee Department of Chemical Engineering, as well as the Centre for Process Systems Engineering at Imperial College London. His work is at the forefront of integrating advanced computer algorithms from optimization, machine learning, and reinforcement learning into engineering systems, with a particular focus on bioprocess control, optimization, and scale-up. Dr. del Rio Chanona earned his PhD from the Department of Chemical Engineering and Biotechnology at the University of Cambridge, where his outstanding research earned him the prestigious Danckwerts-Pergamon award for the best PhD dissertation of 2017. He completed his undergraduate studies at the National Autonomous University of Mexico (UNAM), which laid the foundation for his expertise in engineering.