Abstract
The majority of systems of practical interest are characterized by nonlinear dynamics. This renders the control and optimization of such systems a complex task owing to their nonlinear behaviour. Standard methods of dealing with nonlinear systems such as linearizing around a fixed point may not be an effective strategy for many systems, thus requiring an alternative approach. For this reason, we propose a novel deep learning framework to discover a transformation of a nonlinear dynamical system to an equivalent higher dimensional linear system using data generated from identification experiments. We demonstrate that the resulting learned linear representation accurately captures the dynamics of the original system for a wide range of conditions defined by the training dataset used. As a result of this, we show that the learned linear model can subsequently be used for the successful control of the original system. We demonstrate this by applying the proposed framework to three examples; a benchmark example from the literature, and two practical, complex nonlinear dynamical systems from the chemical engineering domain: the Continuous Stirred Tank Reactor (CSTR) system and finally the four-tank system.
Publication
Computers & Chemical Engineering
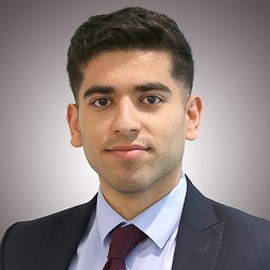
Adaptive Modelling, Control and Optimization of Large-Scale Systems using Machine Learning
Akhil is a PhD candidate working at the intersection of machine learning, optimization and control. He has a proven track record in practical problem solving across diverse industries and domains with research contributions published in various conferences and journals. He graduated with a first class/distinction from the University of Strathclyde in Chemical and Process Engineering and worked as a scientific software developer and engineering consultant before commencing his doctoral studies. Akhil is a member of the Autonomous Industrial Systems Lab supervised by Mehmet Mercangoz and he is co-supervised by Antonio del Rio Chanona.
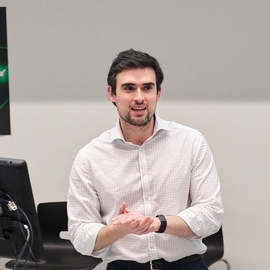
Principal Investigator of OptiML
Antonio del Rio Chanona is the head of the Optimisation and Machine Learning for Process Systems Engineering group based in thee Department of Chemical Engineering, as well as the Centre for Process Systems Engineering at Imperial College London. His work is at the forefront of integrating advanced computer algorithms from optimization, machine learning, and reinforcement learning into engineering systems, with a particular focus on bioprocess control, optimization, and scale-up. Dr. del Rio Chanona earned his PhD from the Department of Chemical Engineering and Biotechnology at the University of Cambridge, where his outstanding research earned him the prestigious Danckwerts-Pergamon award for the best PhD dissertation of 2017. He completed his undergraduate studies at the National Autonomous University of Mexico (UNAM), which laid the foundation for his expertise in engineering.