Abstract
Real-Time Optimization (RTO) plays a crucial role in the process operation hierarchy by determining optimal set-points for the lower-level controllers. However, at the control layer, these set-points may be difficult to track due to challenges in implementation as a result of disturbances, measurement noise, and actuator performance limitations. To address this, in this paper, we present the Adversarially Robust Real-Time Optimization and Control (ARRTOC) algorithm. ARRTOC addresses this issue by finding set-points which are both optimal and inherently robust to implementation errors at the control layers. ARRTOC draws inspiration from adversarial machine learning, offering a novel constrained Adversarially Robust Optimization (ARO) solution applied to the RTO layer. By integrating controller design with RTO, ARRTOC enhances overall system performance and robustness by ensuring the chosen set-points are tailored to the underlying controller designs. To validate our claims, we present three case studies: an illustrative example, a bioreactor case study, and a multi-loop evaporator process. The proposed approach is found to improve RTO objectives, such as profit, by as much as $50%$ in some case studies compared to RTO formulations which ignore the performance of the control layers.
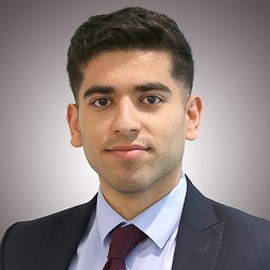
Adaptive Modelling, Control and Optimization of Large-Scale Systems using Machine Learning
Akhil is a PhD candidate working at the intersection of machine learning, optimization and control. He has a proven track record in practical problem solving across diverse industries and domains with research contributions published in various conferences and journals. He graduated with a first class/distinction from the University of Strathclyde in Chemical and Process Engineering and worked as a scientific software developer and engineering consultant before commencing his doctoral studies. Akhil is a member of the Autonomous Industrial Systems Lab supervised by Mehmet Mercangoz and he is co-supervised by Antonio del Rio Chanona.
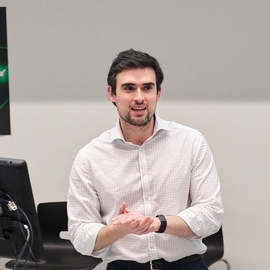
Principal Investigator of OptiML
Antonio del Rio Chanona is the head of the Optimisation and Machine Learning for Process Systems Engineering group based in thee Department of Chemical Engineering, as well as the Centre for Process Systems Engineering at Imperial College London. His work is at the forefront of integrating advanced computer algorithms from optimization, machine learning, and reinforcement learning into engineering systems, with a particular focus on bioprocess control, optimization, and scale-up. Dr. del Rio Chanona earned his PhD from the Department of Chemical Engineering and Biotechnology at the University of Cambridge, where his outstanding research earned him the prestigious Danckwerts-Pergamon award for the best PhD dissertation of 2017. He completed his undergraduate studies at the National Autonomous University of Mexico (UNAM), which laid the foundation for his expertise in engineering.